Exploring the Impact and Future of IA Tech
Delve into the transformative world of IA, uncovering its impact on society and forecasting its remarkable potential for the future.
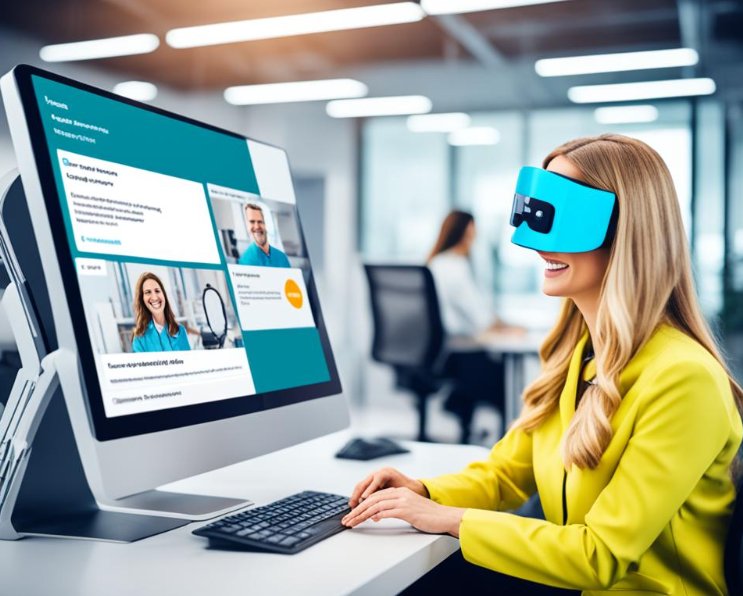
Exploring the Impact and Future of IA Tech
What if the key to unleashing a new era of efficiency and innovation lies not in the "intelligence" of technology but in its "automation"? Welcome to the transformative world of IA—Intelligent Automation. Here, the potential of IA is not just a futuristic dream, but a palpable force shaping the trajectory of industries and societies alike. As we stand on the brink of a technological renaissance, the impact of IA tech reveals itself through smarter processes, enhanced productivity, and unprecedented scalability.
The promise held within IA technologies' future is staggering, yet many are still oblivious to the profound changes already underway. Throughout this article, we will demystify the core aspects of IA, delve into its historical development, and outline the myriad ways it is set to revolutionize our world. Are we ready for the leap from abstract potential to tangible transformation that IA represents? Let's explore.
Key Takeaways
- Understanding the transformative role of Intelligent Automation (IA) in modern society.
- Recognizing the pivotal impact of IA tech on various sectors, driving growth and innovation.
- Unveiling the full potential of IA and the opportunities it presents across industries.
- Analyzing the trends that suggest a vibrant future for IA technologies.
- Setting the stage for a deep dive into the facets of IA that will dictate the next wave of technological progress.
The History and Evolution of IA
The journey from the early conceptual frameworks of artificial intelligence to the sophisticated landscape of Intelligent Automation (IA) we know today has been marked by rapid advancement and groundbreaking innovations.
The Origins of Artificial Intelligence and IA
Tracing the origins of IA begins with a look back at the foundational principles of artificial intelligence. Groundbreakers like Alan Turing, known for the Turing Test, and John McCarthy, who coined the term 'Artificial Intelligence', set the stage for what would become a transformative field. The conceptual leap from AI to IA was rooted in the premise that intelligence could not only be simulated but also applied to automate complex tasks.
Key Milestones in the Development of IA Technologies
The milestones in IA development underscore the remarkable journey from theory to implementation. Key advances include the invention of the perceptron as a model for neural networks, the development of algorithms for machine learning, and the emergence of cognitive computing. These developments were not the work of lone geniuses but the culmination of collaborative efforts from researchers worldwide.
"The evolution of IA technologies reflects a convergence of interdisciplinary research and an unyielding drive towards innovative solutions."
From Academic Theories to Mainstream Adoption
The path from AI to IA adoption wasn't linear; it spread from academic whiteboards and research labs into the real world. As IA became more practical, industries took notice, integrating IA systems to optimize productivity and decision-making processes. What began as complex academic theories morphed into applications that tangibly shift the business landscape.
Decade | Innovation | Impact on IA |
---|---|---|
1950s-1960s | Origin of AI concepts and early neural network models | Laying the groundwork for future IA technologies |
1970s | Expert systems and expansion of machine learning | Providing robust frameworks for IA development |
1980s-1990s | Advances in algorithms and computational power | Elevating the functionalities of IA |
2000s-present | Integration of cloud computing and Big Data | Enabling large-scale IA deployment and sophistication |
Understanding IA: Definitions and Distinctions
In the ever-evolving landscape of technology, the concept and operation of Intelligent Automation (IA) take center stage. As we delve into the realm of IA, it's imperative to grasp and articulate IA definitions, understanding what truly defines this technology beyond the broader spectrum of artificial intelligence. By exploring the AI vs. IA distinctions, we come to appreciate IA's tailored applications and its significance in streamlining complex tasks across a multitude of industries.
What Sets IA Apart from General AI?
Intelligent Automation is notably characterized by its integration of artificial intelligence with process automation. These systems leverage AI's learning and analysis capabilities to drive robots and software that carry out a wide array of routine tasks. This amalgamation of AI's cognitive functions with automation's efficiency gives IA its unique operational edge. Unlike general AI, which aims for a replication of human intelligence, IA focuses on enhancing human productivity through specialized, goal-oriented applications.
Categorizing Different Types of IA Applications
When we consider the various types of IA applications, their categorization underscores the extensive range of solutions IA provides. From data analysis to predictive modeling, and robotic process automation, the applications of IA are diverse, yet unified in their objective to augment human capabilities and increase efficiency. Let's explore some of these applications:
- Data Analysis and Interpretation: IA systems swiftly process large datasets, recognizing patterns and extracting valuable insights.
- Automated Customer Service: Chatbots and virtual assistants use IA to provide real-time, personalized customer interactions.
- Robotic Process Automation (RPA): IA drives robots to perform repetitive tasks with precision, reducing the scope for errors and freeing human workers for more strategic roles.
- Predictive Maintenance: With IA, organizations can anticipate equipment failures and schedule maintenance, ensuring uninterrupted operations.
By acknowledging these categories, stakeholders can make informed decisions about implementing IA solutions tailored to their specific operational needs. As industries continue to grapple with the challenges of digital transformation, understanding the interplay between AI vs. IA distinctions and recognizing the breadth of types of IA applications becomes vital. It's clear that IA defines a new era of optimized workflows, each designed to meet the growing demands of a competitive and technological global marketplace.
The Role of IA in Modern Data Analysis
The era of big data has necessitated a revolution in how information is processed, and IA in data analysis stands at the forefront of this transformation. With the integration of machine learning data processing, IA is able to sift through vast datasets, extracting valuable IA tech data insights that inform strategic business decisions. Let's explore the multifaceted roles of intelligent automation in the realm of data analytics.
IA systems are designed to perform complex analysis at a scale and speed unattainable by human teams. This, in turn, empowers companies to respond to market changes with unmatched agility. Through cutting-edge IA technologies, businesses can harness predictive analytics, which provides foresight into consumer behavior and market trends. The significance of machine learning data processing is especially prominent in sectors where real-time data interpretation can lead to competitive advantages.
- Advanced data visualization tools help stakeholders understand intricate patterns and anomalies within the data.
- Custom algorithms enable businesses to offer personalized experiences to customers, increasing engagement and loyalty.
- Automated reporting features save time and resources, allowing human employees to focus on more strategic tasks.
Below is a comparison of traditional data analysis methods versus IA-enhanced processes:
Criteria | Traditional Data Analysis | IA-Enhanced Data Analysis |
---|---|---|
Speed | Constrained by human capacity | High-speed analysis |
Volume | Limited handling of big data | Efficient processing of vast datasets |
Accuracy | Prone to human error | High precision through algorithms |
Insights | Basic reports and summaries | Deep insights with predictive analytics |
Adaptability | Rigid and slow to adapt | Dynamic and learns over time |
In summary, the implementation of IA in data analysis is not just changing the landscape of data interpretation; it's reinventing it. Through advanced machine learning data processing and the generation of IA tech data insights, businesses are not only making informed decisions but also shaping the future of their industries.
Current Applications of IA in Various Industries
Intelligent Automation (IA) is reinventing service delivery, decision-making, and customer engagement across multiple sectors. Nowhere is this more evident than in the critical realms of healthcare, finance, and retail – industries where IA's precision and efficiency are not just luxuries but necessities for progression and patient outcomes, economic stability, and consumer satisfaction. This section explores the current landscape of IA deployment in these three vital sectors, highlighting specific instances of its transformative impact on both operational processes and end-user experiences.
IA in Healthcare: Improving Patient Outcomes
In the world of healthcare, IA healthcare applications stand at the forefront of medicine's evolution, facilitating early diagnosis, accurate treatment plans, and continuous patient monitoring. By harnessing the power of IA, healthcare providers can offer personalized treatment regimens, improved diagnosis accuracy, and even predict patient health trends, leading to substantial improvements in patient outcomes.
IA in Finance: Risk Assessment and Decision-Making
The financial sector has been particularly receptive to IA in finance, adopting IA tools for more in-depth risk analysis and smarter decision-making. Financial institutions utilize IA to process vast quantities of data for insights that drive loan approval processes, fraud detection systems, and investment strategies, maintaining a steady pulse on market conditions and client profiles.
IA in Retail: Personalization and Inventory Management
Retailers are turning to IA retail personalization to curate unique shopping experiences for their customers. IA's data-driven approach in retail not only anticipates shopper preferences and suggests tailored product recommendations but also streamlines inventory management, ensuring that supply meets the ever-shifting demand of consumer trends.
Industry | IA Application | Key Benefits |
---|---|---|
Healthcare | Patient diagnosis and monitoring | Enhanced diagnosis accuracy and individualized treatment |
Finance | Risk analysis and management | Improved precision in risk assessment and fraud detection |
Retail | Customer experience and inventory optimization | Increased customer satisfaction through personalized offers and efficient stock management |
As IA continues to advance, these industries are reaping the benefits of improved efficiency, enhanced accuracy, and personalized service delivery. The marriage of IA's capabilities with industry knowledge has not only reshaped existing processes but has also paved the way for new service paradigms and business models that seemed inconceivable just a decade ago.
Leveraging Machine Learning for Enhanced IA
The integration of machine learning into intelligent automation (IA) is not just a trend; it is a profound advancement that contributes significantly to IA enhancements. Machine learning IA integration allows for an increase in both the efficiency and adaptability of IA systems, directly impacting the sophistication of tasks such as predictive maintenance, fraud detection, and enhancing customer service.
When discussing the algorithms in IA, it becomes evident that their evolution is critical for handling complex and voluminous data sets. The algorithms, powered by machine learning, are strategically designed to learn from data, identify patterns, and make decisions with minimal human intervention. This symbiosis between machine learning and IA has shown great promise in several areas:
- Operational efficiency through predictive maintenance
- Risk mitigation via advanced fraud detection algorithms
- Heightened customer experiences through dynamic service platforms
IA Function | Machine Learning Role | Outcome |
---|---|---|
Predictive Maintenance | Pattern detection and predictive analysis | Reduced downtime and maintenance costs |
Fraud Detection | Anomaly detection and risk projection | Enhanced security and fraud prevention |
Customer Service | Natural language processing and sentiment analysis | Personalized customer interaction and support |
These advancements are clear indicators that machine learning IA integration is not merely an add-on; it's fundamentally transforming IA capabilities. As industry professionals, it is imperative to not only harness the power of these algorithms in IA but also to innovate continuously to keep up with the rapid shifts in technology.
Indeed, the future of IA shines brightly as these technological collusions pave the way for smarter, more responsive automation that can transcend traditional boundaries and drive industries towards unparalleled growth.
Incorporating Natural Language Processing into IA Tech
The realm of Intelligent Automation (IA) is perpetually evolving, and one of its most impactful strides has been the integration of natural language processing (NLP). By bridging the human language gap, NLP enables IA systems to comprehend, interpret, and generate human language in a way that is transforming the dynamics of communication and data management.
Chatbots and Virtual Assistants: Communication Revolutionized
Incorporating natural language processing in IA has significantly enhanced the functionality of IA chatbots and IA virtual assistants. User interactions are now more intuitive and personalized than ever before, enabling these automated systems to provide immediate, context-aware support and significantly improve customer experience.
Content Generation and Analysis: How NLP Is Shaping IA
The advent of NLP content generation has revolutionized the creation and analysis of content, granting IA systems the ability to craft narratives, summarize extensive documents, and even provide sentiment analysis with remarkable accuracy. This evolution within NLP acts as a crucial catalyst, pushing the boundaries of what IA can achieve in cognitive computing capabilities.
Feature | Benefit to IA Tech |
---|---|
Language Understanding | Enables IA systems to derive meaning from human language inputs |
Conversational Interfaces | Allows for more natural and engaging interactions with IA chatbots and virtual assistants |
Automatic Content Creation | Empowers IA systems to generate human-like text, aiding in content strategy |
Sentiment Analysis | Helps IA systems to understand customer opinions and emotions for better decision-making |
Automated Systems and Algorithms: The Backbone of IA
The intricate grid of IA infrastructure is fundamentally constructed on the robust pillars of automated systems in IA and the role of algorithms in IA. It's the harmonious integration of these elements that automates and streamlines tasks that were once labor-intensive, transforming the landscape of modern industries. Understanding the synergy between these components illustrates how deeply embedded they are in the very fabric of intelligent automation.
Automated systems serve as the executors within the IA framework, carrying out complex sequences of operations without human intervention. This is achieved by implementing sophisticated algorithms which act as the brain behind the automation, enabling systems to function autonomously. These algorithms are not static; they constantly evolve through machine learning, continually refining the automated services provided.
Advanced algorithms facilitate real-time decision-making, accelerating business processes and eliminating the risk of human error.
Over the last few decades, we have observed a monumental shift in how these automated systems have advanced. Thanks to the role of algorithms in IA, industries from healthcare to finance have seen a significant surge in efficiency and accuracy. Their capacity to process vast quantities of data in a splinter of the time it would take a human is nothing short of revolutionary.
- The sophistication of today's algorithms in IA assists in predictive analysis, forecasting market trends long before they become apparent to the human observer.
- Automated systems can preemptively identify maintenance needs in manufacturing, leading to unprecedented uptime and productivity.
Moreover, the robust IA infrastructure is ever-expanding. Its scalability ensures that as a business grows or the scope of tasks becomes more complex, these systems can adapt and manage the increasing load effectively. Productivity soars when automated systems are implemented, freeing human talent to focus on strategic tasks that algorithms are yet to master, such as creative thinking and emotional intelligence.
In conclusion, automated systems augmented by state-of-the-art algorithms are not just complementary features but the mainstay of IA. They signify a tide of innovation that holds the potential to radically redefine efficiency across all spheres. As the role of algorithms in IA becomes more pronounced, we can anticipate not just improvements in the processes but also radical changes in the very nature of work, galvanized by the relentless march of intelligent automation.
Challenges and Ethical Considerations in IA Deployment
As the adoption of Intelligent Automation (IA) continues to surge, addressing the ethical landscape is critical to fostering trust and accountability in IA technology. With a focus on IA ethical considerations, we navigate the complex interplay between innovation and ethical responsibility. Ensuring the integrity and ethical deployment of IA systems necessitates robust engagement with the challenges they present.
Tackling Bias and Ensuring Fairness in IA Systems
Bias in IA systems has emerged as a formidable challenge that could perpetuate and magnify existing societal inequities. IA developers and researchers are responsible for implementing measures to detect and correct biases to ensure fairness and inclusivity in automated decision-making processes. From hiring practices to credit scoring, bias mitigation is imperative to uphold ethical standards in IA technology.
Data Privacy and Security in the Age of IA
IA data privacy and security are at the forefront of user concerns, as they should be. In an era where personal data is a valuable commodity, IA systems must be designed with robust protections to maintain user confidentiality and prevent data breaches. Data custodianship in IA, therefore, requires a delicate balance between leveraging data for innovation and protecting individual rights to privacy.
Establishing Regulatory Frameworks for IA Technology
The rapid evolution of IA technologies has outpaced the existing IA regulatory frameworks. Legislators and regulators are thus challenged to develop agile and effective governance structures that can keep up with technological advancements without stifling innovation. The table below contrasts some of the existing and proposed regulatory approaches, highlighting their attributes and deficiencies.
Region/Country | Current Regulatory Frameworks | Proposed Enhancements | Key Concerns |
---|---|---|---|
European Union | General Data Protection Regulation (GDPR) | Artificial Intelligence Act | Balance between innovation and user autonomy |
United States | State-level regulations (e.g., California Privacy Rights Act) | Federal oversight and standards for IA usage | Consistency and enforcement across states |
China | Cybersecurity Law of the People's Republic of China | Ethics guidelines for IA development | Transparency and international collaboration |
In summary, IA presents a transformative power that, when guided by ethical considerations and supported by vigilant regulatory oversight, can generate a vast spectrum of benefits across various domains. However, as IA systems grow more complex, the imperative to democratize technology responsibly grows alongside. Strategizing to address IA ethical considerations, bias in IA systems, IA data privacy needs, and crafting comprehensive IA regulatory frameworks will remain pivotal in charting the course for the responsible evolution of IA.
Emerging Trends in Cognitive Computing and IA
The realm of intelligent automation (IA) is continually evolving, with cognitive computing trends shaping the forefront of technology. The recent push towards hyper-automation has led to significant breakthroughs, particularly concerning IA predictive analytics and IA decision intelligence. These advancements are not only enhancing current systems but also paving the way for the IA IoT convergence, spawning a generation of smart, adaptive ecosystems.
Next-Generation IA: Predictive Analytics and Decision Intelligence
Driven by an insatiable appetite for data-driven decision-making, industries are leveraging the power of IA predictive analytics to forecast trends and automate complex decision processes. IA decision intelligence complements this by offering robust, strategic frameworks that businesses can use to navigate the ever-changing market dynamics, optimize operations, and deliver enhanced customer experiences.
The Convergence of IA and IoT: Creating Smart Ecosystems
The fusion of IA with the Internet of Things has given birth to interconnected environments that can intelligently interact with users. This IA IoT convergence is redefining how devices operate within the larger Internet ecosystem, making them smarter and more responsive to human needs.
Trend | Application | Industry Impact |
---|---|---|
Predictive Analytics with IA | Forecasting market trends, consumer behavior, and asset maintenance | Retail, Manufacturing, Healthcare |
Decision Intelligence | Strategic planning, risk management, and operations optimization | Finance, Supply Chain, HR |
IA and IoT Convergence | Creating automated smart homes, industrial monitoring, and wearables | Consumer Electronics, Industrial IoT, Wearables |
Conclusion
As we reflect on the transformative role of intelligent automation (IA), one cannot overstate the impact of IA on contemporary technological landscapes and the paths it carves toward the future. The versatile implementations we have explored underscore the technology's potential to revolutionize diverse sectors, from healthcare to finance, and beyond. With each stride in IA technology advancements, it is evident that IA has become an integral part of the machinery driving innovation, guiding industries towards enhanced efficiency and productivity.
The future of IA tech is not merely an extension of its current state but represents an evolutionary leap wherein every aspect of daily life could integrate intelligent automation. Consider the endless possibilities as cognitive computing, machine learning, and advanced algorithms interlace to forge smarter, more adaptive systems. It is within this continuously adapting and expanding realm that innovation in IA shines as a beacon of progress, with the potential to address complex societal challenges and influence decision-making on a global scale.
As the narrative of IA unfolds, the unfolding chapters will be marked by advancements that few have imagined. From scaling new heights in data analysis to nurturing smart ecosystems by merging IA with the Internet of Things, the trajectory is set towards a future where intelligent automation is ubiquitous in its application and impact. In the coming years, observing the harmonious symphony of innovation will not merely be an academic interest but a lived reality, shaping the world as a hub of efficiency, driven by the relentless pace of intelligent automation.
Intelligent Automation (IA) refers to the combination of artificial intelligence and automation, enabling machines to replicate human actions and learning patterns. While general AI aims to create machines capable of performing any human task, IA focuses on automating and improving specific business processes and tasks.
The evolution of AI began with early theoretical concepts in the mid-20th century, progressing through several key milestones such as the development of machine learning and natural language processing. This led to IA, which applies AI to automate complex business tasks and cognitive processes found in various industries.
Key milestones include the creation of machine learning algorithms, the rise of cognitive computing, advancements in data analysis, and the development of automated systems capable of adapting and improving without human intervention.
The integration has revolutionized data analysis by enabling the processing and interpretation of vast amounts of data at speeds and accuracy unattainable by humans. It has empowered predictive analytics and real-time decision-making across various sectors.
In healthcare, IA helps with diagnostic processes and treatment plans. In finance, it improves risk assessment and decision-making. In retail, IA is used for customer personalization and inventory management, among others.
Machine learning and sophisticated algorithms provide the ability for IA systems to learn, adapt, and perform complex tasks with greater efficiency, such as predictive maintenance and fraud detection, thus continually improving performance and outcomes.
NLP is incorporated into IA in several ways, most notably through the development of chatbots and virtual assistants that can understand and respond to human language, as well as through content generation and analysis tools that automate and enhance text-based tasks.
The backbone of IA technology is built on automated systems and advanced algorithms that enable the automation of complex tasks, enhance decision-making processes, and allow IA systems to learn and improve autonomously.
Ethical considerations include addressing bias in IA systems to ensure fairness, upholding data privacy and security amidst widespread data collection and analysis, and establishing robust regulatory frameworks to govern IA deployment.
Emerging trends include advancements in predictive analytics and decision intelligence, as well as the convergence of IA with the Internet of Things (IoT), which is creating smarter ecosystems capable of anticipatory and responsive actions.
Regulatory frameworks for IA are being developed to set standards for the responsible use of automation technology. They aim to protect consumer rights, ensure safety, address ethical issues such as privacy and bias, and foster trust in the technology's applications.
FAQ
What exactly is Intelligent Automation (IA) and how does it differ from general Artificial Intelligence (AI)?
Can you provide a brief overview of the evolution of artificial intelligence leading to the advent of IA?
What are some key milestones in the development of IA technologies?
How has the integration of AI into automated systems changed the landscape of data analysis?
What are the current applications of IA across different industries?
How are machine learning and algorithms enhancing the capabilities of IA?
In what ways is Natural Language Processing (NLP) being incorporated into IA technologies?
What constitutes the backbone of IA technology?
What are some ethical considerations and challenges associated with the deployment of IA?
What are the emerging trends in cognitive computing and IA?
How are regulatory frameworks being established for IA technology, and what is their importance?
What's Your Reaction?
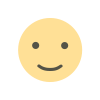
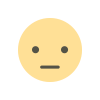
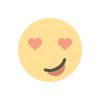
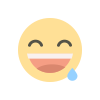
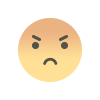
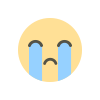
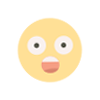